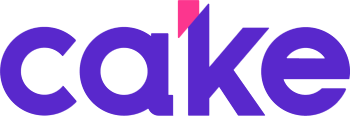
CLIENT CASE Cake
Recommendations model
Ranking the deals list in a relevant and personal way using predictive modeling.

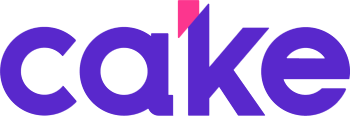
About
Cake
Cake is an award-winning Belgian start-up founded in November 2018 by six senior entrepreneurs with complementary industry experience. It is Cake’s mission to empower
consumers by helping them better understand, manage, and improve their finances. Cake is
already gone through multiple stages of offerings.
Firstly, they were a bank-independent
banking app offering a PFM (personal financial management) product. Integrated into this
app, they provided transaction-based cashback & loyalty offerings.
At the end of 2021, Cake decided to leverage the technology that they built, to fully focus on offering their technology to other financial institutions and businesses.
The services of Cake further developed by offering a data enrichment technology to fully report insights of consumers, the market, and
merchants to retail companies. From that moment they have created a white-labelled version of the transaction-based cashback & loyalty offering and integrated it into the mobile app of Argenta Bank in Belgium.
USE CASE
A recommendation model for Cake
During the research, a recommendation model was set up for Cake whose current list is sorted alphabetically. Cake is offering campaign rewards through the online banking app of Argenta, a Belgium bank. They struggled already a lot of times with ranking these campaign deals as other tasks were more important to this start-up company. Therefore, the research was done for Cake to offer them a first starting point for ordering the campaigns in a relevant way. The purpose is that customers will look at this list and consider more rapidly acting upon those interesting deals that are personally sorted.
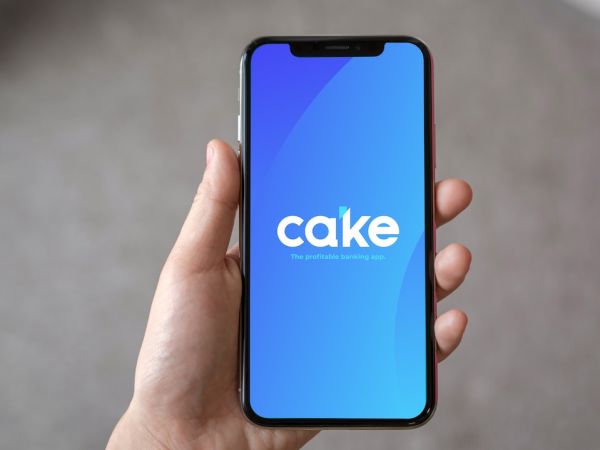
Key points
Data collection
During the data collection phase, we analysed all customer transactional data and selected the most relevant variables to train the model. Our goal is to predict the category in which a customer is likely to make a purchase in the upcoming month. Key variables such as category, merchant, date, and season were carefully considered for their impact on the model's performance.
Data modeling
During the data modeling stage, various models were tested to compare their performance in predicting the category a buyer would likely be interested in next. The best-performing model was then applied to each category, and the predictions with the highest probabilities were used to rank the deals list in Argenta's banking app.
Deliverables
Following the modeling phase, the categories were ranked by calculating the RFM variables for the most predictive categories, focusing on their relevance for the customer's next purchasing month. As a result, customers now see a deal list ranked based on their behavior, rather than being alphabetically ordered.